In order to gauge whether your customers are happy with what you're doing, customer satisfaction is crucial. It is proven that a high degree of satisfaction increases the lifetime value of a customer, more customer retention, and a stronger reputation of the brand. Alternatively referred to as "opinion mining," sentiment analysis can help product managers understand the customer satisfaction level.
Analyzing customer sentiments to predict accurate sentiments has been challenging and time-consuming due to the high volumes of collected data from various sources. Having an NLP and AI expert on hand can help you get started with sentiment analysis if you want to understand your customers in a dynamic market.
What Goes into Procuring Sentiment Analysis
Artificial Intelligence algorithms, Natural Language Processing (NLP) methods, and Machine Learning (ML) models contribute to sentiment analysis. This includes neural networks, unigram algorithms, skip-gram algorithms, and bag-of-word regression models using machine learning and deep learning (DL). The use of natural language processing techniques in machine learning software allows unstructured texts to be sorted by emotion and opinion.
As an example, let's look at this. Imagine that you are responsible for understanding the general sentiment in the support conversations at your company. Get a sense of your customers' ongoing mood and tone. You would need an emotion classifier in such a situation to produce predictions regarding relevant conversations. Dashboards that are often designed to measure customer satisfaction can be populated with sentiment data you've collected from your customers.
Why is Sentiment Analysis Important in Business?
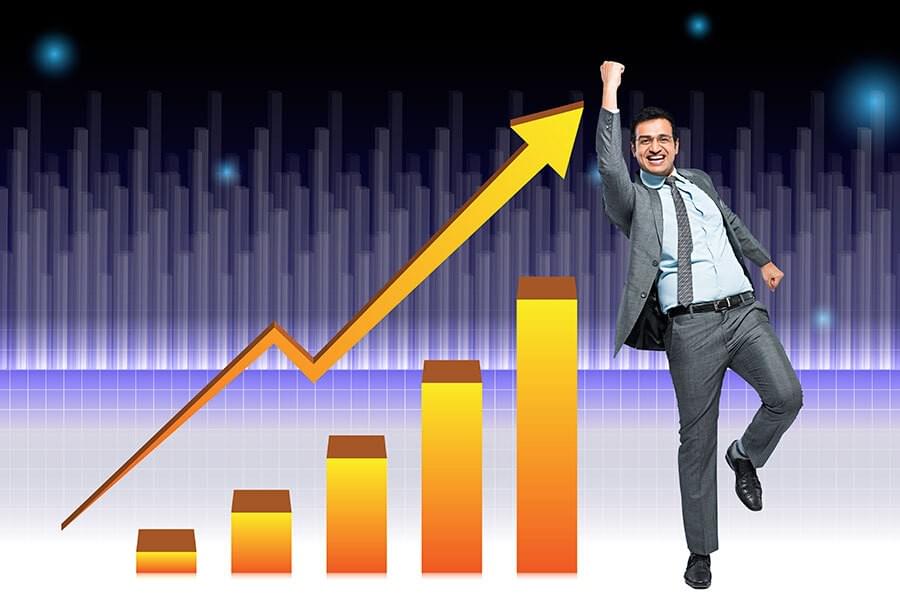
At first glance, it may seem like a fancy class project, but sentiment analysis has many practical applications in the business world. Using sentiment analysis to solve business problems is an excellent starting point.
1. You can aggregate customer sentiment from free-form feedback data and identify the primary promoters or detractors of your product or service. Once the detractors have been converted to promoters, you can gradually implement corrective measures to rebuild trust.
2. It is possible to detect hateful and inappropriate comments on your online platform, which will help you keep it free of bullies.
3. The feedback you receive from recent employees, peer reviews, and manager feedback can help you identify demotivated or about-to-quit-employees and provide them with constructive ways to succeed.
Based on these sentiment analysis examples, you can see that sentiment analysis is an excellent tool for gaining a better understanding of employees and customers, keeping platforms safe, improving customer shopping, and learning from competitors. Sentiment analysis can be combined with other artificial intelligence technologies like text summarization to provide deeper, more powerful insights.
Industry Applications of Sentiment Analysis
In sentiment analysis, the text is analyzed to identify and extract subjective information, which businesses can use to measure how customers feel about their service offerings. Using AI-driven technology, sentiment analysis traces the undertone of text by channeling extensive digital information.
The use of sentiment analysis is widespread across various industries. Several popular sentiment analysis applications exist, such as monitoring social media, managing customer support, and reviewing customer feedback. Here are a few more to get you going with the idea of integrating sentiment analysis into the marketing & customer analysis symmetry of your business:
1. Social Media Monitoring
Social media posts often contain some of the most honest opinions about your products, services, and businesses because they’re unsolicited. By incorporating NLP experts on board, you can analyze individual emotions and overall public sentiment across all social platforms based on their comments, feedback, and reviews.
In chat, sentiment analysis can detect sarcasm, understand acronyms (OMG, LOL, ROFL, etc.), correct misspellings and misuses, and detect sarcasm.
2. Managing & Monitoring Business Reputation
Sentiment analysis is widely used in business for brand monitoring. A business can leave negative reviews online for a long time, and the longer it does so, the worse the situation will become. Negative brand mentions can be detected as soon as they occur with sentiment analysis tools.
Furthermore, you can monitor your progress over time by keeping track of your brand's image and reputation. You can use this information to develop usable statistics and information about your brand, whether you are monitoring news stories, blogs, forums, or social media for information about your brand.
In addition to following trends and anticipating outcomes, machine learning can be relied upon to stay one step ahead of the competition and become proactive rather than reactive.
3. Analyzing the Market Response to a Product
Learn what users are saying about your new product soon after launch, or examine feedback you may have yet to see. Aspect-based sentiment analysis allows you to locate only the information you need based on your search terms (interface, UX, functionality). Identify what makes your most valuable customers happy, learn how your product is perceived by your target audience, and improve your product. The whole thing is based on sentiment analysis.
4. Get to Know the Voice of Your Customers (VoCs)
Creating a personalized customer experience requires communication and listening to the voice of your customers. As a result, businesses have access to a large number of conversation sentiment analysis datasets that can be integrated into the development of ML models in order to automate the sentiment analysis process. Taking all feedback, you receive from customers, such as web feedback, surveys, chats, and customer service calls, and evaluating it. This data can be categorized and structured with the help of sentiment analysis so that you can discover recurring topics and concerns.
5. Analyzing Market & Competitors
In order to research the market and your competitors, you can use sentiment analysis. Get a sense of who your competitors are mentioning positively and how your marketing efforts compare. Look at how your competitors speak positively to their customers, and take some of those phrases and use them in your own brand message and tone of voice guide.
Advantage of Manual Sentiment Analysis Over AI-Integrated Machines & Apps
In a world of billions of people, each communicates uniquely, e.g., adding tiny variations to the language and attaching sentiments to it, which are easy for us to understand but difficult for machines to understand. Sarcasm, for instance, is the opposite of what is true when we use it in our sentences. Sentiment analysis through machine learning models is difficult to predict customer behavior, as a matter of fact. Therefore, manual sentiment analysis has an advantage over automated sentiment analysis performed through AI-integrated applications and machines.
Final Words
For accurate sentiment analysis results, a blended approach to sentiment analysis is the most effective way for businesses to utilize it to understand customer behavior. Consequently, Cogito, an NLP expert, combines manual and automated approaches for accurate sentiment analysis.
In addition to manually conducting sentiment analysis of your social media channels and customer feedback on other platforms, the experts here can also assist you in getting AI training data in order to build ML models for automated sentiment analysis based on real data rather than assumptions made based on a small sample.
This post is originally published at click here